
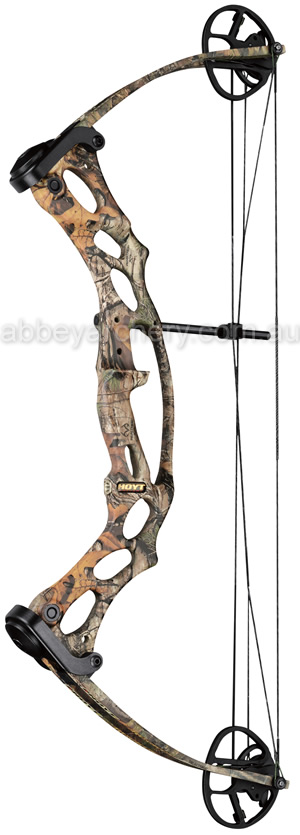
Furthermore, by effectively recovering the inherent noise structure, LbC leads to highly robust models.įigure 1 provides an illustration of a simple 1D regression experiment using a single-layer neural network with 100 neurons and rectified linear units (ReLU) nonlinear activation. This eliminates the need to construct priors on the expected residual structure and makes it applicable to both homogeneous and heterogeneous data. More specifically, LbC uses two separate modules, implemented as neural networks, to produce point estimates and intervals, respectively, for the response variable, and poses a bilevel optimization problem to solve for the parameters of both the networks. Though calibration has been conventionally used for evaluating and correcting uncertainty estimators, this paper advocates for utilizing calibration as a training objective in regression models. For a confidence level α, we expect the interval to contain the true response for 100 × α% of realizations from p( x). Using a large suite of use-cases, we demonstrate the efficacy of our approach in providing high-quality emulators, when compared to widely-adopted loss function choices, even in small-data regimes.īuilding functional relationships between a collection of observed input variables x = )\), the intervals are considered to be well-calibrated if the likelihood matches the expected confidence level. We propose Learn-by-Calibrating, a novel deep learning approach based on interval calibration for designing emulators that can effectively recover the inherent noise structure without any explicit priors. Popular choices such as the mean squared error or the mean absolute error are based on a symmetric noise assumption and can be unsuitable for heterogeneous data or asymmetric noise distributions. In this work, we study an often overlooked, yet important, problem of choosing loss functions while designing such emulators. Consequently, there is a recent surge in utilizing modern machine learning methods to build data-driven emulators. Predictive models that accurately emulate complex scientific processes can achieve speed-ups over numerical simulators or experiments and at the same time provide surrogates for improving the subsequent analysis.
